North York, ON M2J 5B4
The advancement of AI writing tools is increasing at a very fast pace; we have a situation where artificial intelligence can write as human beings do. Whether it is writing a novel or preparing a business report, AI systems such as ChatGPT can perform the work at par with, or even better than, human beings.
But this impressive capability presents a tricky challenge: how can we ensure that we are able to distinguish the text generated by an AI tool from a text generated by a real person? This means that, as algorithm-generated text becomes more and more complex, the distinction is becoming increasingly blurred. This has big implications for many industries that involve writing as a central means of operation.
In this article, we focus on the main difficulties and nuances of the distinction between artificial and human intelligence when it comes to content and writing. We'll explore the critical role of a trusted AI detector for content validation and its potential to address these pressing concerns.

The Evolution of AI Writing Technologies
To understand the difficulties in identifying AI content, it's important to first recognize the accelerated progress of underlying AI text generation systems:
Early Machine Learning Models. The antecedents to today’s systems used machine learning techniques such as recurrent neural networks to process big data of written human language. Though in its early days it was as simple as the word predictor and the ability to recognize patterns, the groundwork of the algorithm was built.
Large Language Models and GPT-3. In 2020, OpenAI introduced GPT-3, which utilized a dataset and model architecture to generate fairly cogent text, thereby opening up more creative possibilities. This “large language model” approach led to a lot of innovation in a short period of time.
Fine-Tuning and Customization. Once the large pretrained models started being offered through APIs, developers could adapt them to specific niches. This in turn fuels even more focused AI writing for certain content types and for content themes.
Emergence of Meta-Learning. Now the algorithms can learn about themselves in a way that allows them to become more specialized much faster with fewer examples, which reduces the need for the Big data sets. This combination of learning and meta-learning enhances the AI’s writing proficiency.
As these technologies continue advancing rapidly, identifying AI content is becoming exponentially more difficult over compressed timescales. Evaluating writing is now enormously more complex than just a few years ago.
Key Challenges in Distinguishing AI Content
There are a few core challenges that make evaluating whether content is AI-generated or human-written so problematic:
AI Can Mimic “Human” Style and Voice
Prior AI writing tools were not very efficient in emulating voice, tone, and other subtleties of the writing style. But now, large language models can replicate the way humans use words, construct sentences, tell stories and other latent features that we attribute to ‘human-like ‘writing. This mimicry poses fundamental problems in AI detection based on the analysis of the writing style.
No Systematic “Tells” or Giveaways
It was previously thought statistical analysis could catch subtle “tells” revealing AI provenance, like higher lexical diversity or more consistent section lengths. But such systemic aberrations are fading away as models better analyze and replicate human writing conventions across diverse datasets. There are fewer giveaways to leverage.
Customization Enables “Domain Expertise”
Fine-tuning approaches allow AI models to digest niche datasets—from scientific papers to movie scripts—enabling domain-specific content. This means AI tools can now exhibit subject matter expertise across different fields that humans spend years acquiring. Detecting deficiencies in factual accuracy or topical mastery is no longer a reliable indicator.
Rapid Pace of Technological Change
Because underlying models leverage interdependent algorithms evolving at exponential rates, evaluation benchmarks struggle to keep pace. Metrics and approaches that reveal AI content today may be obsolete in 6–12 months as capabilities advance. The technologically moving target makes continuous detection adaptation essential—but intensely challenging.
These factors underscore why distinguishing AI writing has become immensely problematic. But additional qualities render evaluating applied contexts even harder.
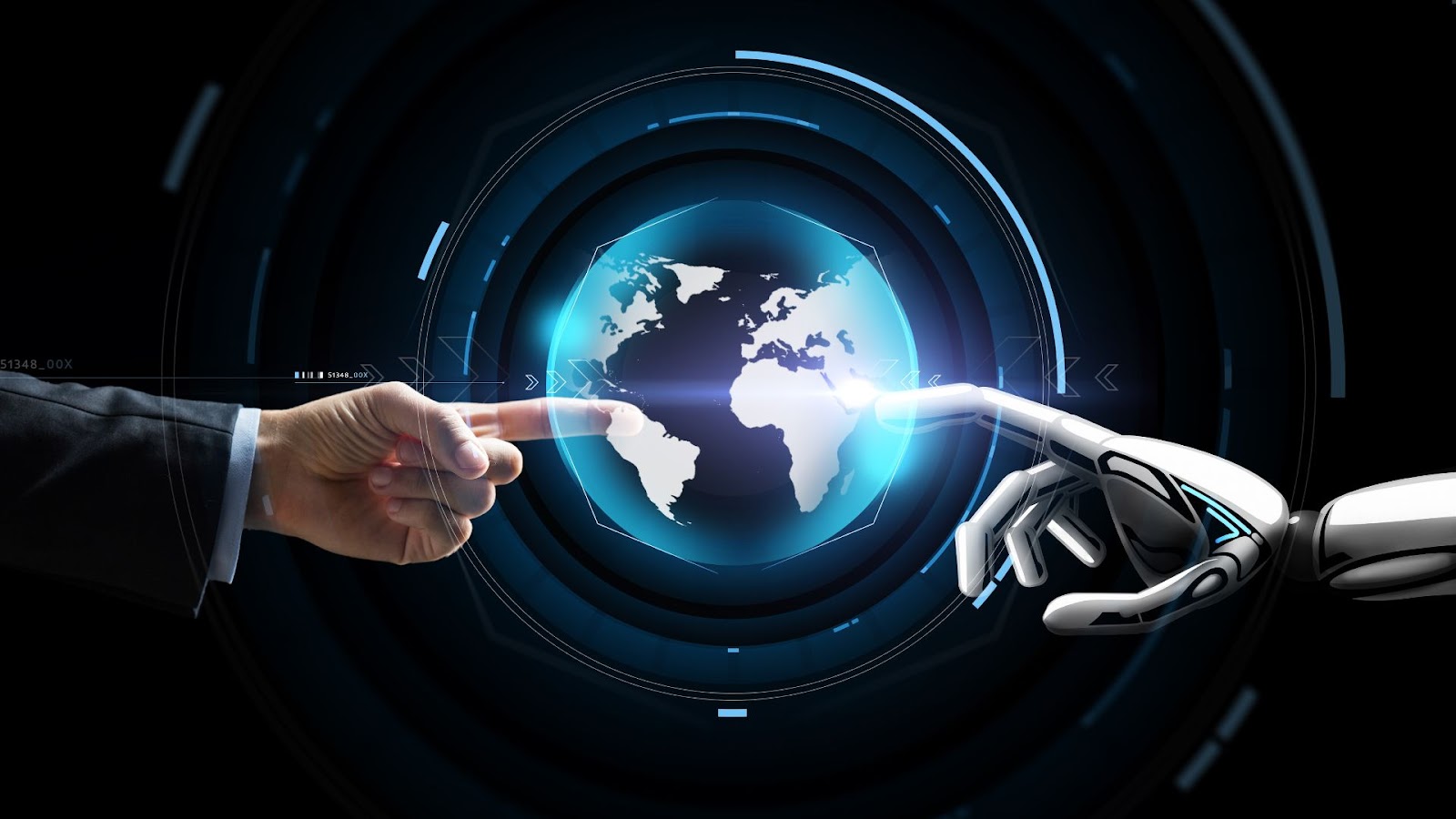
Applied Challenges in Real-World Detection
Beyond fundamental technical obstacles, several practical realities further frustrate applied efforts to differentiate human and AI content:
Lack of Insight into Origins
In real situations, you rarely have transparency into a text’s provenance, which introduces guesswork. Does unconventional phrasing reflect AI imperfections or human creativity? Without insight into the underlying source, ambiguous indicators get interpreted subjectively.
Economic Incentives Around AI Content
As high-quality AI writing gains traction, economic upside may incentivize creators to misrepresent AI origins to boost perceived value. Detecting when financial motives could inspire “AI washing” creates another dimension of uncertainty absent from technical benchmarks.
Blending of AI Assistance and Human Curation
In practice, writing workflows involve collaboration between AI tools and people. This interplay—with humans editing or building on AI drafts—blurs lines around provenance and introduces organic hybridization where both contribute. Untangling these integrated contributions poses enormous difficulty.
Constant Evolution of Workflows
Just as core technologies continue advancing, real-life application workflows harnessing them also rapidly evolve. Emergent hybrid approaches fuse new creative dimensions—from AI verse refined by poets to academic studies built on algorithmic literature reviews. Such continuous workflow innovation makes benchmarking a moving target.
These practical challenges compound the overarching complexity of gauging AI's creative frontiers as technology and adoption evolve.
Looking Ahead: Ongoing Struggles to Distinguish AI Content
As revealed by the complex technical and applied realities discussed above, it only means that the task of defining AI writing will be even more challenging in the future. Several fronts will be central to this continued challenge:
Core Technology Advancements
The increasing speed and frequency of algorithmic advancements in large language models due to data and computing power growth will extend the creative possibilities of AI writing systems. And each wave of progress will continue to push the differences between AI and human work to the level where it becomes nearly impossible to distinguish between the two.
Adoption Dynamics and Applications
With the enhancement of quality and reduction of cost, AI writing will seep into more areas—from productivity tools to business solutions. Increasing real-world application will lead to specialization and commercialization, which make the applied detection even more challenging due to the use of steganography and deception.
Coevolution of Detection Methods
Approaches to identifying AI content will certainly progress through enhanced linguistic analysis, statistical benchmarks, and evaluation of error modes. However, the interplay between advancing generation and detection capabilities will likely produce arms races across domains.
While differentiation struggles will persist, encouraging developments like transparency frameworks and testing methodologies focused on ethics and accuracy can help guide responsible innovation. But in terms of pure technical capacity, the lines between artificial and human writing will only grow hazier as this AI-fueled creative revolution propels forward. The challenges ahead are profound, as are the possibilities.
Conclusion
From stylistic imitation to the incorporation of AI-generated work into work processes, the identification of AI-generated content raises monumental and increasingly complex challenges. At the center of this growing identification problem is the existence of large language models that keep on learning at exponential rates. Evaluative activity is hampered by both fundamental and practical challenges in all contexts.
But while technical capability soars past human-comparable levels on shorter cycles, there is the need for AI. Making things transparent, assessing things righteously, and permitting scrutiny offers society and markets an opportunity to adjust. Still, in the creativity aspect, artificial writing intelligence is already breaching limits many thought would take decades to achieve—and the curve is steep. The scope of this change is going to be phenomenal—so will be the consequences.
Please fill out the form below if you have any advertising and partnership inquiries.